Home > Columns > Executive Interviews
Executive Interview
Tobias Goebel - Hands-on Product Marketing Leader,
Can we build machines that understand us?
Tobias Goebel, 6 Mar 2020
The question of whether we can build machines that truly think
is a fascinating one. It has both practical and philosophical implications, and
both perspectives answer a key question very differently: “how
close to the real thing (human thinking) do we need to get?” In fact - does
rebuilding the exact human ways even matter? And are we too easily impressed
with anyone claiming they have accomplished this Franksteinian feat?
From a purely practical perspective, any machine that
improves a human task on some level (speed, quality, effort) is a good machine.
When it comes to “cognitive” tasks,
such as reasoning, or predicting what comes next based on previous data points,
we appreciate the help of computer systems that produce the right outcome
either faster, better, or more easily than we can. We do not really care how
they do it. It is perfectly acceptable if they “simulate”
how we think, as long as they produce a result. They do not actually have to
think like we do.
The question of whether machines can truly think has become
more relevant again in recent years, thanks to the rise of voice assistants on
our phones and in our homes, as well as chatbots on company websites and
elsewhere. Now, we want machines to understand — arguably a different, more
comprehensive form of thinking. More specifically, we want machines to
understand human language. Again we can consider this question from two
different angles: the practical, and the philosophical one.
John Searle, an American professor of philosophy and
language, introduced a widely discussed thought experiment in 1980, called The
Chinese Room. It made the argument that no program can be written that, merely
by virtue of being run on a computer, creates something that truly is thinking,
or understanding. Computer programs are merely manipulating symbols, which
means operating on a syntactical level. Understanding, however, is a semantical
process.
Searle concedes that computers are powerful tools that can
help us study certain aspects of human thought processes. He calls that “weak AI”. In his 1980 paper, he
contrasts that with "strong AI”: “But
according to strong AI, the computer is not merely a tool in the study of the
mind; rather, the appropriately programmed computer really is a mind, in the
sense that computers given the right programs can be literally said to understand
and have other cognitive states.”
Cognitive states are states of mind such as hoping, wanting,
believing, hating. Think (sic!) about it: proponents of strong AI, and they do
exist, claim that as soon as you run an appropriately written computer program
(and only while it is running), these computers literally are hoping, are
wanting, etc. That surely must be a stretch?
Searle’s thought
experiment is summarized by him as follows:
“Imagine a native
English speaker who knows no Chinese locked in a room full of boxes of Chinese
symbols (a data base) together with a book of instructions for manipulating the
symbols (the program). Imagine that people outside the room send in other
Chinese symbols which, unknown to the person in the room, are questions in
Chinese (the input). And imagine that by following the instructions in the
program the man in the room is able to pass out Chinese symbols which are
correct answers to the questions (the output). The program enables the person
in the room to pass the Turing Test for understanding Chinese but he does not
understand a word of Chinese.”
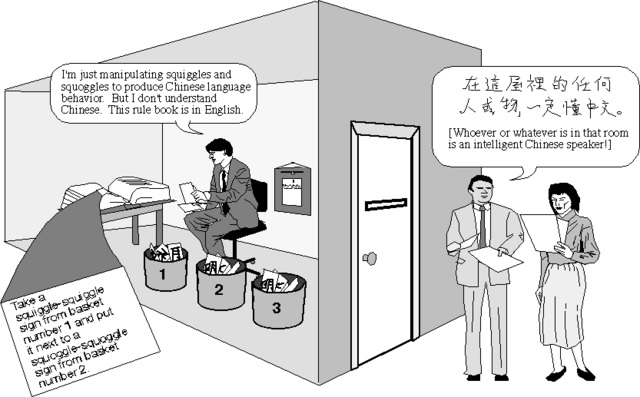
This is a simple but powerful thought experiment. For
decades now, other philosophers have attempted to shoot holes into the
argument, e.g. claiming that while the operator him- or herself might not
understand Chinese, the room as a whole actually does. Yet all of these replies
are eventually refutable, at least according to Searle, so the argument is
being discussed and studied to this day.
Strong AI is of course not necessary for practical systems. As an excellent example of that, consider the social chatbot Mitsuku. (A “social bot” has no purpose other than to chat with you, as opposed to what you could call functional or transactional chatbots, such as customer service bots.) Mitsuku is a five-time winner (and now a Guinness World Record holder) of the Loebner Prize, an annual competition for social bots. She is entirely built on fairly simple “IF-THEN” rules. No machine learning, no neural networks, no fancy mathematics or programming whatsoever. Just a myriad of pre-written answers and some basic contextual memory. Her creator is Steven Worswick, who has been adding answers to Mitsuku since 2005. The chatbot, who you can chat with yourself, easily beats Alexa, Siri, Google, Cortana, and any other computer system that claims it can have conversations with us. (Granted: none of the commercially available systems do claim that social banter is their main feature.) Certainly, Mitsuku by no means aims to be an example of strong AI. It produces something that on the surface looks like a human-to-human conversation, but a computer running the IF-THEN rules is of course nowhere near a thinking machine. This example, however, shows that it neither requires a machine that “truly thinks”, nor a corporation with the purchasing power of an Amazon, Apple, or Google, to build something that serves a meaningful purpose: a single individual with a nighttime and weekend passion can accomplish just that. And Mitsuku, with its impressive ability to chitchat for long stretches of time, is meaningful to many, according to the creator. |
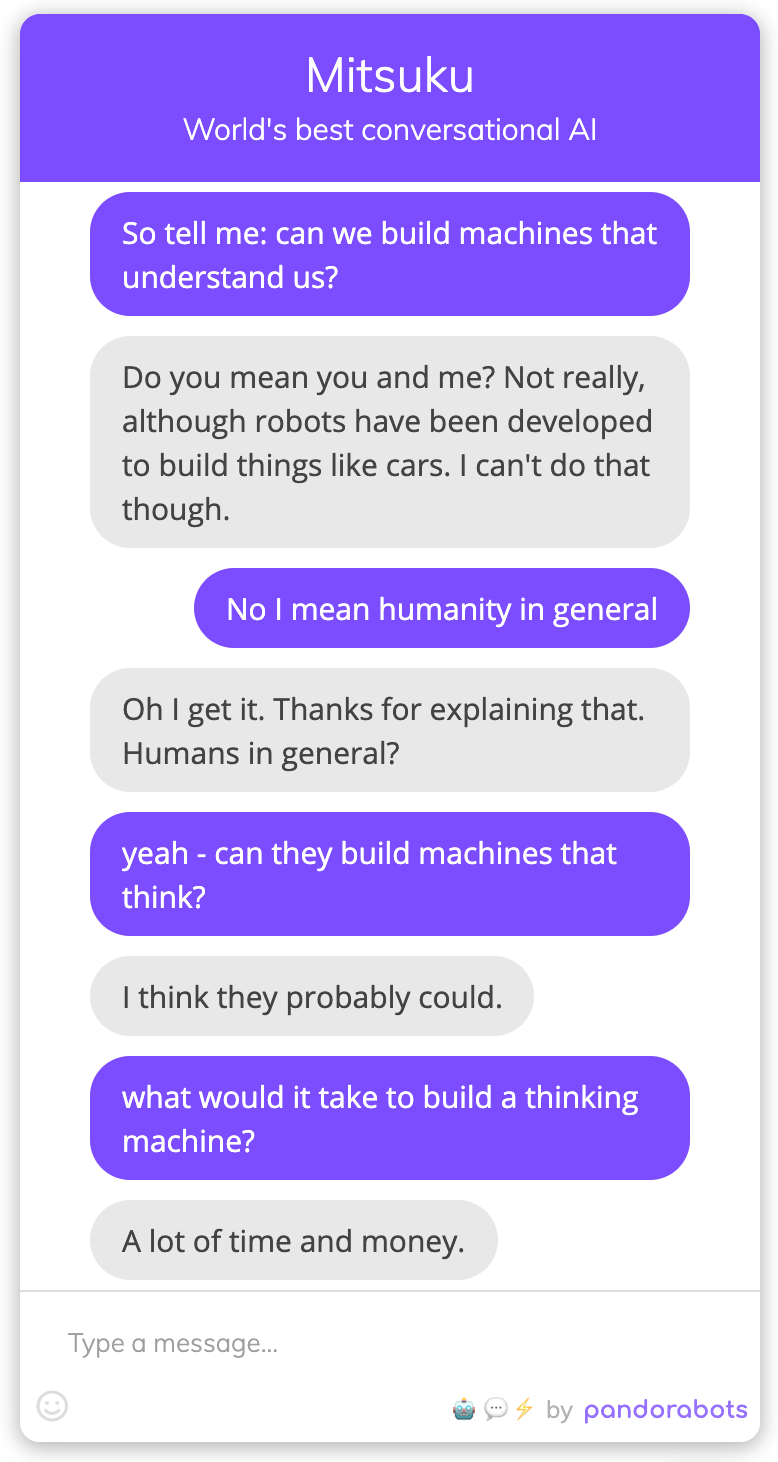 |
It is easy to get distracted by technological advancements
and accomplishments, and the continuous hype cycles we find ourselves in will
never cease to inspire us. But let’s make an attempt to not let them distract
us from what fundamentally matters: that the tools we build actually work, and
perform a given task. For chatbots, that means that they first and foremost
need to be able to have a meaningful conversation in a given context. Whether
they are built on simple rules or the latest generation of neural network
algorithms shouldn’t matter. Despite that concession, it will probably remain
forever human to marvel at advances towards solving what might be the biggest
philosophical question of all: can we ever build a machine that can truly
understand?