Home > Columns > Executive Interviews
Anthrolytics Executive Interview
Jonathan Hawkins, co-founder, Anthrolytics
Click image below to view the ebook
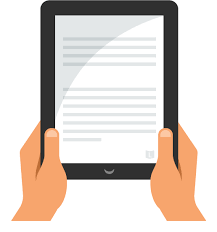
Sheri Greenhaus, Managing Partner of CrmXchange, discussed Anthrolytics’
SaaS solution with Jonathan Hawkins, co-founder, Anthrolytics Ltd. Anthrolytics
combines behavioral and data science to predict what a customer will do next,
and why.
Sheri Greenhaus: Please give us an overview of your
solution.
Jonathan Hawkins: The genesis of our solution stemmed from a
major bank's inquiry into the likelihood of their customers leaving if they did
something unpopular, such as increase prices. This is a question many
organizations have pondered at some point. The answer however, partly depends
on the customers' feelings towards the brand. If customers love the company, a
minor misstep will probably be forgiven. On the other hand, if they already
dislike the brand, it could be the final straw prompting them to leave.
So, the real question became: How can we gauge our
customers' feelings towards our brand?
Traditionally, people have relied on customer surveys, speech analytics, and
other similar methods to try and gauge the mood of customers. However, these
methods have limitations. They often rely on direct interactions, and for a
major bank with millions of customers, only a small percentage responds or
contacts the customer service center annually—usually less than 5%. Moreover,
these methods offer lagging indicators, meaning they might capture a customer's
positive experiences from over a year ago, despite recent negative experiences.
Employee surveys in contact centers face similar issues.
People tend to be honest about their feelings only when they are about to
leave. My co-founder developed algorithms that focus on "moments that
matter," understanding that behaviors result from the cumulative impact of
many experiences over time. It's usually not a single big event but a series of
small incidents that affect loyalty or lead to resignations, unplanned
absences, or customer churn.
To address this, we use existing operational data,
particularly in contact centers. We create emotional profiles for agents daily,
determining their moods and categorizing them into empagraphic segments
(cohorts of employees that have similar sets of feelings), which are then linked
to behaviors. Some agents may be at risk of resigning in the next 90 days (with
varying degrees of probability), while others might be likely to to take
unplanned absences or see a fall in performance. Others might be highly
motivated and engaged. We are currently focusing on contact centers due to
their high attrition rates. For instance, a center with 60% attrition among
10,000 agents or 40% out of 50,000 agents – staff attrition and absence in the
U.S. costs companies $100 millions each year.
In reality, almost every agent will eventually leave; the
question is when. Our solution empowers team leaders to understand their
agents' daily emotions, who's at risk of unplanned absences or resignation and
why. The goal isn't to eliminate attrition but to keep those predicted to
resign for a few more months. The ROI is remarkable. In a 1,000-seat contact
center with 20% attrition, it typically costs $6 million annually in management
time, lost productivity, recruitment, training, and onboarding. By retaining
employees for just two more months, companies can save around $1 million
annually, contributing directly to the bottom line.
When people consider contact centers costs, they often
overlook the hidden costs, like recruitment and training expenses. We can help
mitigate part of that issue. The harder-to-quantify aspect is employee
wellness. Research shows that mental health issues, primarily stress and
burnout, account for 62% of long-term sickness. While we're not offering a diagnostic
or therapeutic model, if we can observe an employee's mood declining, we want
to intervene for their well-being. A recent study reported that 87% of
employees appreciated being treating empathetically and that in turn drives improved
loyalty and productivity.
Today, people struggle because, until we introduced
Predictive Behavioral Analytics, they had no way to understand how employees
truly felt at scale. Empathy requires this understanding, and remote and hybrid
work environments have made it even more challenging. The days of walking
around a call center looking for actionable insights are gone. We utilize data
companies already possess, such as WFM and ACD data, and leverage it in a
unique to predict resignations, unplanned absences, and motivation levels.
Sheri Greenhaus: Most people don't grow up aspiring to be
contact center agents. What we have found through our webinars, is that those
who are agents often look for a path to advance within the organization. Providing
a path for agents within the organization may also help to retain them. Are
there particular industries that you work with?
Jonathan Hawkins: We work with any organization that
operates a contact center, typically those with multiple hundred agents or
more. So, it encompasses a wide range of organizations, industries, and
territories.
We find that many BPOs need to demonstrate to their clients
that they are effectively managing their programs. Employee wellness may be a
genuine concern for some, while others see it as a necessary requirement. We
enjoy working with companies that recognize the significance of employee
well-being, because that is where we can have the greatest impact.
Sheri Greenhaus: You mentioned core factors that people care
about. Could you categorize the top 5 most important aspects overall?
Jonathan Hawkins: Certainly, while there may be variations
depending on the company and industry, broadly speaking, you can group them
into three buckets: 1) shift time, 2) type of work, and 3) time off.
Sheri Greenhaus: Are there specific requirements, such as
speech analytics, needed to use your solution?
Jonathan Hawkins: There are no specific requirements for
speech analytics. Our focus is on predicting likely behavior based on
individuals' experiences with the company. While some outliers, like personal
issues at home, may exist, our goal is to provide valuable data within the
systems that organizations already use.
Unlike other software companies that create proprietary
dashboards and struggle to get users to adopt them, we take a different
approach. Our belief is that users want valuable data integrated into their
existing systems. For example, we integrate with performance management
systems, providing an employee wellness section alongside traditional contact
center performance metrics. Team leaders can now have a holistic view within
their existing engagement systems and identify employees at risk of unplanned
absences or other issues. This approach enables more effective team management,
especially in remote work scenarios where identifying potential problems
becomes crucial.
As an operational level, you can consider the center as a
whole. For example, if 15% of individuals appear to be at risk of taking
unplanned absences, how does this affect workforce management and productivity?
Now, consider if it's 10% or 20% of people who seem likely to resign within the
next 90 days. How does this impact our recruiting efforts? One particularly
valuable aspect is evaluating the overall motivation and wellness score of the
center as a whole, and I'm currently reporting this as a single number within
the range of -100 to +100.
Do we need to implement management changes? If I'm looking
at this from a historical perspective, and I have one team, but everyone's
performance seems to be declining, is it the manager or the work environment?
So, now we can start examining factors like work type, call type, shift type,
and manager type for various users. The key point we were discussing is
integrating all of this into existing platforms.
Sheri: Let me ask you this: if I've just purchased Verint
performance management and I'm using your solution, will it appear that I'm
fully utilizing Verint's solution?
Jonathan: Yes, the way we're currently integrated, we are
one of their marketplace partners. We have the capability to effectively
integrate with any system due to our flexible architecture. We can take data in
flat file format, process it within our platform, and return it in the desired
format for their use. There's no one else offering a solution like ours –
simple to use and understand, powerful in its impact.
Sheri Greenhaus: People will inevitably leave regardless of
our efforts; the goal is to extend their stay. With your solution, when you
identify a group likely to leave within the next two months, does it enable
better recruiting planning?
Jonathan Hawkins: Absolutely. If we can predict resignations
in the next 30, 60, or 90 days, and let's say our training program takes an
average of 8 weeks, we can plan ahead. For instance, if we anticipate 200
resignations within 90 days, factoring in 60 days for training and 30 days for
recruitment, we can begin recruiting immediately. By intervening with team
leaders, we might retain some employees for an additional month or two. This
way, we can adjust our recruiting strategy accordingly, and it aids in our
forward-looking planning.
Traditional employee surveys can't provide this level of
insight; they offer lagging indicators, not leading ones. My focus with AI is
on providing organizations with leading indicators for effective workforce
management and employee well-being while maintaining operational efficiency.
Without effective management, we won't have the resources to sustain our
business in the long run.
The reality for most organizations is that they will
inevitably have to do some things that upset employees from time to time, such
as asking them to work over a public holiday, or take a late night shift. What
we give those employers is the ability to see which employees are likely to
take that badly so that they can take proactive steps to secure the employees
willing cooperation.
Sheri Greenhaus: Could it also involve identifying the 50
out of those 200 employees that the company wishes to retain? Then, the company
could determine their specific needs, such as career progression or flexible
schedules, and focus on retaining them.
Jonathan Hawkins: I fully agree. We had a similar situation
a few months ago with a BPO company that lost a contract and needed to reduce
its workforce. They viewed it as natural attrition to save costs, avoiding
layoffs. So, absolutely, we can help with that. Identifying individuals for
fast-tracking and investing in their development is crucial. If we understand
what motivates them, we can allocate resources accordingly, nurturing the next
generation of leaders who have experience on the front lines.
Moreover, understanding the factors driving positive behavior,
such as work type, shift type, and their combinations, can be integrated into
the recruitment process. If we know that certain roles require specific traits,
we can begin screening for those early on. However, it's important to note that
we don't have the capacity to diagnose mental health issues, and we don't
handle the recruitment side of things.
But perhaps the biggest difference in our approach is that
it considers each employee as an individual – all of whom have had different experiences
of work, even if they do share some common characteristics. This is fundamental
to delivering an empathetic experience – understanding the wants and needs of
each employee.
Sheri Greenhaus: What about on the customer side? We've been
talking about the employee, right? What is the ability to predict what
customers are going to do?
Jonathan Hawkins: The system was originally built for
customer attrition. The initial use case we had was for one of the top three
banks in the U.S. with tens of millions of customers, and these are the people
who asked us, "If we do something our customers don't like, can you do a
better job of predicting how likely are they to leave?" That was the
genesis of the idea on the customer side.
We said to the bank, "Okay, here's a cohort of people.
Forget about your demographics. Forget about your typographic segmentation.
Here's a group of people who have a high propensity to leave because they just
don’t like you. And here's a practical idea: rather than trying to sell to
everybody in the same demographic (you and I may be in the same financial
demographic, but I may hate the brand, and you may love them), why not target
people like Sheri and sell to them because your conversion rates will be much higher?"
What they saw by doing that very simple thing was an increasing conversion rate
of 18%. But also, interestingly, a reduction in marketing costs of 38% because
they weren't selling to people who didn't want to buy.
Sheri Greenhaus: Is this for new or existing customers?
Jonathan Hawkins: It was actually cross-selling to existing
customers. Our two areas of focus are the contact center and existing
customers.
If you think about this concept of behavior being the result
of a cumulative impact of experiences, what that means is you can now start
tracking people on a graph effectively. So, as an existing customer, I've had
this experience, then that one, and the other one, and the next. And I start
tracking the impact of all of those experiences. What's interesting is if you
now start segmenting those customers before they hit the threshold where
they're likely to churn, you can put loyalty campaigns in place before a
customer even thinks of leaving. You can track this path and build a cohort of
people who are potentially at risk before they even realize it themselves. That
case study with the bank was fascinating. They also saw a reduction in churn in
the segment we were looking at, over 11%, because they were able to intervene
very early. Everything we do allows people to proactively intervene to change
behavior and make a difference whilst it is still relatively cheap and easy to
do so.
In another case, we worked with a relatively small telco, with
around 4 million customers. We conducted a proof of concept with them,
implementing A/B testing. They operated in an emerging market characterized by
a pay-as-you-go environment where people often had multiple SIM cards and
bought data from various providers based on their preferences.
They ran consistent outbound campaigns to upsell their
services. Initially, their approach was to target customers based on classic
demographic segmentation - who had the money to buy, rather than who was most
likely to buy. We provided predictions that pinpointed a cohort of individuals
we believed were not only most likely to purchase but also able to spend the
highest amounts.
What's particularly intriguing is that their average revenue
per user had been stagnant, fluctuating slightly above and below the baseline.
On a scale where 0 was the baseline, they occasionally achieved a modest +8%
increase, but often dipped below it.
Once we implemented the campaign, focusing on the targeted
group within the A/B test, they experienced an impressive 89% increase. There
were two main factors behind this success: Firstly, they were now selling to
people who genuinely wanted to buy from them because the customers liked them –
a seemingly simple but powerful concept. Secondly, these individuals were
economically active and willing to spend more.
Additionally, beyond the financial gains, the most valuable
insight they uncovered was that they had been investing substantial amounts in
improving their network infrastructure. This investment was based on their
belief that network quality was the top priority for their customers, as
indicated by customer surveys.
However, during our initial calibration study at the
project's onset, we discovered that this specific cohort of customers didn't
prioritize network quality. Surprisingly, their primary concern was their
ability to purchase data for friends and family. The company had implemented a
data usage limitation within their app, and by removing this restriction, they
witnessed a significant increase in spending. This success was the result of
selling to the right audience and continuously adapting to their needs.
Furthermore, it highlighted that much of their previous
survey data might have been biased, as respondents tended to be individuals who
were dissatisfied with their services. People often ask us if we utilize social
media data, but we generally avoid it due to its extreme polarization. Social
media feedback tends to come from those who either absolutely love or loathe a
brand, making it less valuable for gaining nuanced insights.
We've got a proof of concept running at the moment with some
financial institutions, which I find really interesting. We're predicting
likely customer behavior, which is being integrated into another platform, for customer
journey orchestration. So, as you hit the customer journey orchestration,
instead of pushing everyone down a predetermined path, like, "This is the
best way for people to buy," you can now say, "Okay, well, Jonathan
is a high-value customer from a customer lifetime value perspective, and
Jonathan's really unhappy" — that's the prediction. So, actually, what I
want to do is, rather than push Jonathan down an automated route, I want to
connect Jonathan straight to live agents in the contact center.
I think as these things evolve, when we start having
intelligent routing in contact centers, you can start thinking about this type
of technology as a front-end. So, when somebody hits the contact center, how do
I route them most appropriately? If we think Jonathan's happy and going to buy,
well, why don't I just push Jonathan through self-service? If Jonathan might
have an issue with loyalty, well, actually, I probably want him to speak to an
experience employee with high EQ. So, you can start to get very intelligent to
maximize customer service through hyper-personalization, to a degree. Its
hyper-personalization based on how I feel about you and how I treat you empathetically.
Even with a chat, if I know how somebody feels when they interact with the
chatbot, with the advent of Generative AI, I can dynamically change the content
to be empathetic.
Sheri Greenhaus: Is there anything that we didn't touch on
that you would like our audience to know?
Jonathan Hawkins: I think the key things for me really are
empathy is a message. You've got to understand how people feel, but it's such a
significant untapped resource. Whether you're thinking about the customer side
or the employee side, the ROI is untapped and spectacular. That's probably the
biggest message — that by doing this, even if you're only improving by 5%,
you're still going to make money on it. It's a no-brainer.